This transcript has been edited for clarity.
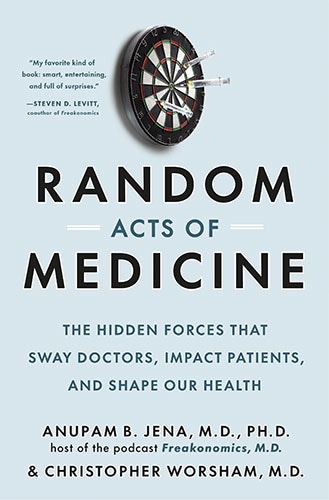
Eric J. Topol, MD: Hello. This is Eric Topol, here with my co-host, Abraham Verghese, and this is the Medicine and the Machine podcast. We're pleased today to have as our guest Bapu Jena, a physician and economist at Harvard who has written, with his colleague Christopher Worsham, a fascinating new book called Random Acts of Medicine. Bapu, welcome.
Anupam B. Jena, MD, PhD: Thank you for having me.
Topol: This is a very interesting book. I think the whole medical community, no less the public, will find it extraordinarily interesting. You start off with what you call a natural experiment. Some people will say, "Oh, that's just an observational study." Can you tell us what you mean by "natural experiments" and how they differ from — or are potentially better than — randomized studies?
Jena: Randomized experiments are quite common in medicine. When someone takes a drug, if we're lucky, it's been subject to a randomized trial, where investigators randomly assign a group of people to one drug, and another group to another drug or a placebo. That allows us to say something about the causal effect of getting that drug, because everything else is being held constant by virtue of the randomization.
The opposite of that is what we think of as a straightforward observational study, in which you look at people who take that drug in the real world, and you compare them with people who take the other drug. You hope that the factors that led them to take the drug are not correlated in some way with the outcome of interest, and that any differences are related to the mechanism of action of the drugs.
That usually is not the case because selection is involved. People choose to take certain medications — or someone recommends a medication to them — for a host of reasons, some of which a researcher can observe but many of which a researcher cannot observe. We call that confounding. That's why observational studies are not often relied upon by the US Food and Drug Administration.
Now, there's something in between, which we call natural experiments — a scenario where nature essentially randomly assigns people to one intervention vs another. We use the term "quasi-randomized" because it doesn't occur at the hands of an investigator. With this method, we think we're getting closer to the causal effect of the intervention.
It's observational in the sense that we are looking back at data that already exist. That part is true. But it's not the same as an observational study because we're taking this issue seriously, that the way a person happens to be given an intervention in the real world is typically not random. So, we want to find situations where it is as good as random.
Abraham Verghese, MD: I want to say how much I enjoyed your book. To me, it was a succession of fascinating stories. I'm marveling at the way your mind works. It made me think of this not very well-known tradition of MD economists. We had Alan Garber here as our colleague for a while, and then he had a cadre of MD-PhD economists. You're clearly in that tradition. Talk a bit about what led you into getting an MD with a PhD in economics.
Jena: That was actually quite random. I'd spent some time in college working in a basic science lab, and I wanted to do an MD and a PhD in something like immunology or cell biology. But I also studied economics in college. I thought to myself, Wow, I'm applying to medical school. I need to round out my background a little bit and study a "humanity." Economics is about as far from the humanities as I could think of, but that was my logic at the time.
Fast-forward a few months when I'm applying to medical schools. I visited the University of Chicago, and the director of that MD-PhD program noticed that I had studied economics. He asked me, in sort of an offhand way, "Would you want to do your PhD in economics instead?"
That's how it happened. It was sort of random in that respect, and it's taken my life down a completely different path from where it otherwise would have gone.
Topol: My favorite chapter was on left-digit bias. In fact, the name of the chapter is "What Do Cardiac Surgeons and Used-Car Salesmen Have in Common?" The car is priced $1 less, illustrating left-digit bias.
You presented many graphs of what has been known as discontinuity, whether it's bypass surgery, kidney transplants, or opiate prescribing; one after another, you show this left-digit bias. Can you talk about that? How come we're so stupid?
Jena: I think it's human nature. Humans aren't perfect and doctors are humans, so there's the transitive property — doctors aren't perfect.
At the grocery store you see a bag of Doritos priced at $1.99. The reason it's $1.99 is because the mind looks at that leftmost digit (a "1") and that seems cheaper than $2.00, even though it's only a penny cheaper. You don't see stores, for example, relying on pricing of $1.63 vs $1.64 to try to shift consumers to purchase a product. It's something specific about that left digit.
It's not surprising that you could trick a human being into purchasing a bag of Doritos at the margin that they may or may not have purchased had the price not been $1.99. What surprised me, though, is that you would see the same sort of thing happening in a high-stakes decision, one that is arguably well thought out and for which the implications are enormous.
In the book, we talk about some of our own work, which looked at people who came to the hospital with a heart attack. And for some of these people, cardiac bypass surgery makes sense. We show that if you happen to come to the hospital just a week shy of your 80th birthday — let's say 79 years and 50 weeks old — you are more likely to receive cardiac bypass surgery than if you came 3 weeks later, when you are 80 years and 1 week old.
This occurs because the doctor looks at the patient and says, "They're in their 70s" or "They're in their 80s." The older patients are, the less likely doctors want to do invasive things to them. That was our finding. It is interesting to me because it shows that the same sort of behavioral heuristics that apply in other parts of our lives also happen in high-stakes settings.
It also shows us how behavioral economics can create these quasi-experiments where people are, by chance, randomized to undergoing coronary artery bypass graft surgery or not. Then you can look at the outcomes a year later and see what happens.
Topol: At many points in the book you talk about the features that define best-performing physicians. I'd like you to comment on two of these. And then later we'll get to the cardiology convention.
Can you comment on your findings about women and age, and the differences between hospitalists and surgeons where older may be better?
Jena: Sure. Let me start with the age study. If you're a patient and you see a young doctor walk into the room, you might be concerned that the young doctor doesn't have a lot of experience in the practice of medicine.
If I'm being honest with myself, as I think about trying to find medical professionals for my family or friends, I'm not typically looking at doctors who are fresh out of residency training or fellowship. I'm looking years out because I have the perception that experience matters. But ultimately, that does become an empirical question.
It's not an easy one to solve, because if patients believe that older, more senior doctors are more experienced and will provide better outcomes for them when they are particularly sick, who are those doctors going to attract in the real world? They're going to attract patients who are sicker. So, you can't simply look at older vs younger doctors and say anything about the causal effect of being seen by one or the other.
You must construct a scenario where people are seen by older or younger doctors by chance. That's where the hospitalists come in. In that scenario, if you have a 45-year-old doctor who works on Mondays, a 35-year-old doctor on Tuesdays, and a 65-year-old doctor on Wednesdays, that's all random. The patient going to the hospital with chest pain or shortness of breath doesn't go there knowing that the 35- or the 65-year-old doctor happens to be in the hospital that day. It's random.
That allows us to uncover a causal effect, and we see two things. One is that the older the doctor is, the worse the outcome of 30-day mortality. How likely are you to survive 30 days after that hospitalization?
That effect starts pretty soon after residency. It's not as if we're comparing 80-year-old and 35-year old internists who work in the hospital. For every 5 years beyond residency, you see a bump in mortality.
But the benefit is preserved among higher-volume doctors. If you're an older doctor and you still maintain a high volume of patients, you don't seem to pay that cost that comes with the older doctor with a low patient volume. So, there is a silver lining when it comes to the internists.
For surgeons, we find the opposite. The older surgeons tend to have better outcomes. We're focusing on emergency surgeries, where we think that the allocation of the patient to the doctor is kind of random.
Topol: That's important because it demonstrates how you drill down on things. You don't conduct only one layer of analytics; you keep going and going. That was a theme throughout various chapters in the book.
Verghese: That hospitalist story was painful to read, but I knew it was true because I attend less and less often. I'm aware that I'm not as up on the hospital protocols as my junior colleagues, so I'm reaching out to them all the time. But I had the illusion that perhaps I brought a level of wisdom that keeps bad things from happening to patients when doctors order things that patients don't need — diagnostic tests and so on. But the finding about age rings true, even though I didn't want to accept it.
Jena: One thing we didn't do in our study that we should have done is look at rarer diseases and more uncommon presentations of common diseases, where you might see experience shine a bit more brightly. Whereas if we're talking about management of more routine problems, where technology or science is evolving quickly, the older doctor might not be up-to-date. That's a different ballgame.
Verghese: On that hospitalist finding, did you match their volume of patients? When you're a young hospitalist, you take a lot of shifts — many more than I'm willing to take — and as you get older, you might take fewer shifts because you have children and other obligations. Did you match for the number of patients seen?
Jena: Yes, exactly, and we show that if you focus on the higher-volume older hospitalists, they do just fine, because they continue to maintain that volume.
Another piece that we published, in JAMA Internal Medicine a couple of years ago, is not in the book but conceptually it's very similar. We looked at doctors who were primarily clinically focused vs people like me, who spend some time clinically but spend a lot of time doing other stuff. On average, the clinically focused docs tend to have better outcomes, meaning lower mortality. Again, that's not surprising. It is not true for every single doctor, but on average that's what you see.
Topol: Can you talk about the advantage of women physicians?
Jena: I want to warn everyone first, before getting to the more controversial study. Eric, to your point, we recognize that some of these findings will be controversial, so it is important to do as much as we can, use all the bells and whistles, to make sure that what we're finding is credible. In that same chapter, we looked at the outcomes between male and female doctors.
We have done a lot of work in the past looking at gender differences in promotion and pay. We've shown that, even when you account for otherwise similar work — we're looking at people who are similarly productive clinically and from a research perspective — men are promoted faster and they are paid more for equal work.
That question hasn't been looked at as much in the clinical context, meaning, what are the outcomes of women doctors vs men doctors? Again, we have that problem that the types of patients who see women vs men as doctors may be different. So, again, we rely on this hospitalist idea that sometimes by chance patients are seen by men vs women doctors.
We found that the mortality rate for patients who, by chance, are seen by a female hospital physician is lower 30 days after hospitalization than for those seen by a male physician. There are reasons that may be true, none of which we can pin down. It could be differences in the time spent by women doctors with their patients compared with men. A paper in The New England Journal of Medicine suggests that may be true, at least in the outpatient setting, and might generalize to the hospital setting.
It may be related to differences in clinical reasoning or diagnosis. Outside of medicine, in investments, for example, there's good evidence that men tend to be more risk-averse. So, is it the case in medicine that male doctors might hone in on diagnoses prematurely or faster than a female physician would? If so, you could imagine that there would be higher rates of misdiagnosis. A lot of things could explain the difference.
The take-home, of course, is not that we should take all male doctors and make them female. It's also part of a broader social commentary: Women doctors are doing quite well, if not better. But we also need to figure out what it is that women are doing differently that can be replicated by everyone — both male and female doctors.
Verghese: I wrote a paper about a year ago, with a colleague from Amsterdam; the title was "Medicine Is Not Gender-Neutral — She Is Male." Our point was that our construct of physicianhood, for centuries, has been a male construct. Only recently have we achieved this equality, with more women trainees in medicine than men.
But the metrics we consider to be part of physicianhood are all male derived, and some of them may be detrimental. The rising to the top and the power-consolidating protocols are the opposite of nurturing humanism. When we wrote that paper and came across these studies showing that women do better, I was gratified, because I think it validated the touchy-feely side of medicine that increasingly, in our obsession with data, we leap right over.
Jena: That's a good point. I'm an economist, so I think a lot about things like cost. If you look at American medicine, cost is the thing that most health policy scholars talk about. But even in situations where people don't face any direct medical costs — maybe the medication is free — we still observe that they adhere to the medication regimens only about 50% of the time.
So, what gives? Why is it the case that if something is free and we believe it's beneficial for their health, they don't adhere to it? I believe that's where some of these "touchy-feely" things may come into play because it is also about trust.
If I don't perceive there to be a need, personally, for me to take this medication, or if I don't trust the messenger, I may be less likely to take it. So, to the extent that we see differences between male and female physicians — as we have seen in adherence to medications by their patients — if that's a function of some of these softer attributes that probably have hard importance, that's something we need to know more about.
Topol: You also touched on better adherence to guidelines. But the reflection about more time spent with patients may go along with Abraham's allusion to more compassion that is generally seen. Obviously, there is no shortage of exceptions, but maybe that's a general trend.
Now, I want to get to the cardiologists because I have an interest. It's a fascinating chapter. So, every year, particularly years ago, all of the leading cardiologists would flock to the American Heart Association (AHA) meeting. It's not so much the case these days. You studied that and found that you're a lot safer if you go to the hospital during the AHA convention. Can you tell us about that?
Jena: First of all, I thought the opposite would be true. I remember being a trainee around the time of one of these big meetings — either the AHA or American College of Cardiology (ACC) — and it felt like the staffing was different. So it put this idea into my head: I wonder what happens to people who have acute cardiac problems when cardiologists are away at these big meetings?
If you look at the data, you see that hospital mortality, or 30-day mortality, after coming in with a cardiac arrest or very severe heart failure is lower during the dates of these meetings. This is a good natural experiment. I can say that conceptually because people don't choose to have their heart attacks when the AHA and ACC are holding meetings. They probably have never heard of those organizations, much less choose to have their heart attacks when those meetings are taking place.
It's totally random, and you can see that in the data. The characteristics of patients are just like those in Table 1 of a randomized trial. They're as good as random — similar during meeting dates and non-meeting dates. So, the mortality difference is coming from behavior changes of the cardiologists who remain behind.
The other data point was that rates of certain procedures — we focused on stenting because that's something we can measure quite well — fell by about 30% during the dates of the meetings. Imagine you have two people. One is a 40-year-old construction worker who has chest pain when he's working on the construction site. He goes to the emergency department (ED). He gets an EKG. He has had a heart attack, confirmed with some lab tests. He gets a stent and goes home in 2 days and does well.
The second person is a 90-year-old woman who has 10 different medical problems. She has the exact same chest pain at her nursing home. She is brought into the same ED. Her EKG shows the exact same changes as the first guy. Her lab tests look exactly the same as the first guy. She also gets a stent, but she dies within a week or two of the procedure because of complications.
That story would be well understood by most people, and what it gets at is that we often perform medical care as if it's black and white. Sometimes it is black and white, but other times it's quite gray, or it could even be black and white in the other direction. We just don't know. Obviously, cardiologists aren't intentionally trying to harm 90-year-old women who are coming in with chest pain. But it's the desire to help, the desire to do something, that sometimes backfires if the risk/benefit is not favorable for that type of person.
Verghese: Has anyone ever looked at the first month of internship and what that does to mortality in academic medical centers? My theory is that it's safer. I used to attend often during that month, and I felt like everybody was much more wary.
Jena: There are data. Unfortunately, they don't line up with what we would hope. They call this the "July effect," and we've looked at this in cardiac care. If you look at people who happen to be hospitalized in July in academic medical centers vs in May or June, and you do the same thing in nonacademic medical centers as a control group, you tend to see that mortality is higher in July.
But it's not a uniform finding, Abraham. Sometimes you see it, sometimes you don't. Our insight was that maybe we are looking in the wrong place. You can't just look at the typical hospital patient because it takes a lot to go wrong for them to be harmed and die as a result of that care. But if you look at high-risk cardiac patients, even small errors might matter. And we see large July effects as a result.
But what you're saying is exactly right. The care patterns do change. Physicians are aware of the problem and so they adjust to it. If they hadn't adjusted, I believe that the mortality effect would be even larger.
It gets to the same idea that, on a rainy day, you would think that traffic mortality would typically go up because the rain makes it harder to see and the roads are more slippery. But there's also a compensating effect, which is that fewer people go outside in the rain, and they're also aware that it's raining. It's when you're not aware of the underlying threat that you're particularly susceptible to a problem, but if you're aware of it, you can adapt. It may not offset the primary effect, but it at least compensates.
Verghese: I'm curious about your mechanics of writing this book. Writing any book is an architectural challenge and you're doing it with a co-author, but it came off beautifully. I love the book. Talk a bit, if you would, about the process.
Jena: For years now, I've been thinking about writing something, in part because a lot of this work is approachable. You don't have to be in medicine to understand the underlying thought experiment that is at play here. I was trying to think of a way to take these kinds of studies, which I think people would be interested in or learn something from, and put them into a book.
This is not a collection of short stories. We tried to do something more than that. We tried to take a finding and then expand on it to ask, what does this teach us about how healthcare works, when it works poorly, and how it could work better?
My co-author, Chris Worsham, is a critical care doctor and health policy researcher at Harvard. I met him 5 or 6 years ago, around the time I was thinking about writing a book. I knew I didn't want to do it alone, so I asked Chris if he would do this with me. He had similar interests in this style of thinking, and it came together nicely.
Topol: That's terrific. A critical care physician certainly adds another dimension, with all of the in-hospital factors you were analyzing.
The last chapter is about politics and how that influences many of the biases that you articulate throughout the book. Can you give us a sense about the overall impact of politics, particularly in the United States?
Jena: We saved the most controversial topic for last because this has obviously been a heated issue in the past few years. We start by talking about some of the studies on how politics could influence medical care, which it does in a lot of ways. For example, we talk about what happens when healthcare systems are acquired by Catholic hospital systems and how the type of care that's offered changes. So, that's a way in which religion or politics could permeate how care is provided.
We spent some time in the book on one study where we had information on US physicians' political donations because those are in the public record. We linked the data and looked at end-of-life care provided by Republican and Democratic physicians. These were patients who died in the hospital or shortly thereafter. We looked at whether they were more likely to be in the ICU, to be mechanically ventilated, or to have feeding tubes placed. We didn't find any evidence that Republican and Democratic doctors treat patients any differently at the end of life, which is a reassuring finding.
Then we switched gears to COVID-19. We intentionally did not speak much about the pandemic in the book. But we mention a few different studies, one of which I conducted. We were interested in the question of whether small social gatherings early in the pandemic, with people you knew and trusted, were a driver of disease spread.
That was a hard question to study. Think about the type of data you would need for hundreds of thousands, if not more, people. You would need to know when they were gathering and whether they got COVID-19, which are both big data problems. You can't get that kind of data. You would also need to know whether there were other things they were doing that were different. People who gather might be more likely to travel, less likely to wear masks, and all sorts of other behaviors.
This is how we got around that: One reason people might gather is for a birthday. If you look at people, in any given city, in which a household has a member with a birthday, that household is about 30% more likely to have a COVID-19 diagnosis in the 2 weeks following that birthday than an otherwise similar household, in that same city, in that same week.
We looked at hundreds of thousands of households. This was a natural experiment because birth dates are random. There's no reason that a household with a birthday in it would be more likely to get COVID-19 unless they were gathering for that birthday. The effect — what I'd call a birthday effect — was stronger in households in which a child had a birthday.
The politics angle was as follows: We found that this birthday effect was identical in highly Republican and highly Democratic counties. That was interesting, because in the beginning of the pandemic and even throughout, there's been this polarized discussion over how Republicans and Democrats differ when it comes to public health behaviors: masking, social distancing, vaccine adoption — whatever. Is what people say in surveys or on social media what they actually do?
When you look at the behaviors here, we saw very similar patterns between Republican and Democratic counties, at least in this one domain. It changes, though, with vaccines. In the book we talk about a study by Jacob Wallace and others at Yale. When the vaccines came around, we started to see a divergence between Republican and Democratic areas of the country. It's a complicated story, but the way I'd put it together is that the differences we talked about, and continue to talk about, in the pandemic are there but they're probably much less than the similarities that all groups have shared. We just tend to focus on the differences, which I don't think makes a lot of sense and doesn't do good for policy.
Verghese: Where do you look for your data, and what kind of databases are you using? What's the engine behind all of this? I could ask the question but I'd have no idea how to go about looking for birthdays and COVID and so on.
Jena: Good question. There are a couple of engines. One engine is the idea. I always think that's the most important part. I had an idea about a birthday and COVID-19. So where do we go?
When a person sees a doctor, the doctor will bill the insurance company for that care. That information is recorded by insurance companies. If a patient goes to the pharmacy and fills a prescription for a medication, and the insurer pays for that prescription, that information is recorded by the insurance company. So, insurance companies have assembled voluminous and comprehensive data that allow us to know something about the medical care that people are receiving; what kind of medications they're taking; what kind of doctors or hospitals or therapists they're going to; and what they're being diagnosed with.
That data is all anonymized. I can't look up information on a particular person. It's not like an electronic health record.
Statistical work, or "econometrics," go into all of these findings. Most of what we talk about in the book, and most of what I do, doesn't require any fancy statistical work, which I think is a virtue, because when I see a paper that says that this drug is associated with this condition or this side effect, and the statisticians account or correct for a number of different factors, that gives me pause.
I view these variables — what we call confounders — almost like pests or rodents. If you see one, you know there are others. You just don't see them, but they're there. And economists have long appreciated that. That's why we focus on randomization.
For all the studies I do, or try to do, I want them to have a simple interpretation, which is, if people are quasi-randomized to this or that intervention, what is the outcome? And then, can we attribute it to that difference in what they're exposed to?
Topol: Even in randomized trials, the baseline characteristics are hardly ever completely balanced among the two or three groups. And then you have this cumulative effect of small differences that may not be statistically significant, that are like rodents. Before we wrap up, you didn't get to it in the book at all, but because this is the Medicine and the Machine podcast, I want to get to the machine angle. As you allude to, MD can stand for "make diagnosis," and we know that there are lots of errors and human biases.
A promise out there is that maybe artificial intelligence (AI) can help with these things, by working around some of the biases we have, some of the things we've been reviewing during this conversation. Have you thought about how we can go from random acts of medicine to AI-supported, better practice of medicine with fewer errors?
Jena: There's a lot of promise, and it probably comes in three different flavors. One is a data flavor. With all of the available data about people, when a person walks through the door, a computer or machine should be able to synthesize information that a physician or another practitioner will not be able to see because it's not available to them in their record or they don't have the time to process that information. So one benefit of AI is to be able to cull all of this information together in a way that is not possible for the physician.
The second flavor is that, in areas where pattern recognition is important, such as EKGs, I think there is a big scope, and it happens in two ways. One is that it helps doctors identify patterns that we know exist but that the individual doctor has failed to see. For example, if someone has chest pain, they get an EKG and the EKG demonstrates that this person is having a particular type of heart attack. If the findings are a little subtle, the cardiologist or the doctor may not pick up on it. So that's a misdiagnosis, even though the pattern was there and another doctor might have picked it up.
But there's a separate space where AI can come in, by establishing patterns that are new to us as human beings and doctors, that we don't know exist because we know what we know. There are other features of EKGs. If you took a million people who have EKGs performed, and you look at those who had heart attacks and those who did not, there are other signatures in those EKGs that we, as humans, don't know to pick up on. The machine can do that. I believe there's a lot of value for AI in predicting diagnoses.
The third flavor is how critical diagnosis is in medicine. Doctors often have a narrow set of diagnoses that they work with and are familiar with. At minimum, a computer could say to the doctor, "Here are five different diagnoses that you haven't considered."
And the doctor can look at it and say, "I think this is good (or bad) — check, check, no" and then proceed forward. But at least they're presented with that information to be able to say, "All right, I agree or disagree with this," and then they move on. There's a lot of opportunity there.
Verghese: I must say, it's been a wonderful experience to read the book and also to chat with you. I can't wait to see what natural experiments you have, or will have, your eye on. Thank you so much for joining us.
Topol: Congratulations to you and Chris on your book. It's a gift for the medical community.
Follow Medscape on Facebook, X (formerly known as Twitter), Instagram, and YouTube
Credits:
Image: Penguin Random House
Medscape © 2023 WebMD, LLC
Any views expressed above are the author's own and do not necessarily reflect the views of WebMD or Medscape.
Cite this: Left-Digit Bias and Other Random Acts of Medicine - Medscape - Sep 07, 2023.
Comments